Event History Analysis with Stata
by Hans-Peter Blossfeld, Katrin Golsch, Götz Rohwer
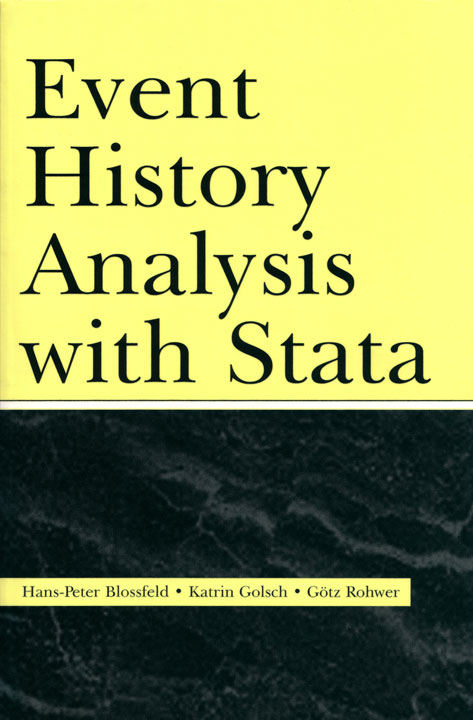
Event History Analysis with Stata, by Hans-Peter Blossfeld, Katrin
Golsch, and Götz Rohwer, presents survival analysis from a social
science perspective. Introducing the mathematics and statistics of
survival analysis, along with substantive discussions of social science
data–specific issues, the authors give examples throughout using Stata
(version 9) and data from the German Life History Study. The text
covers both basic and advanced topics, from an introduction to life
tables to fitting parametric models with unobserved heterogeneity. The
authors aptly illustrate the entire research path required in applying
event history analysis, from the initial problems of recording
event-oriented data, to data organization, to software applications, to
interpreting results.
Chapters 1 and 2 introduce event history data, discussed substantively,
and the data structures used to contain them. Chapter 3 introduces
nonparametric descriptive methods including life tables, product-limit
estimation of the survivor function, and comparison of survivor
functions.
Chapters 4–8 focus on estimation using parametric survival functions.
This section discusses not the usual exponential, Weibull, etc., models
but rather issues such as period-specific effects, qualitative and
quantitative covariates, time-dependent covariates, and multiepisode
data.
Chapter 9 discusses the Cox proportional hazards model, whereas chapter
10 covers problems with parametric model specification, including
unobserved heterogeneity.
The book has a parametric model focus, which for some readers will be a
strength and for others, a weakness. For the latter group, the weakness
is minimal because the coverage of the Cox model is adequate given the
foregoing discussion.
Event History Analysis with Stata is aimed at the professional social
scientist but could also serve as a graduate-level text. A website
providing supporting materials for the book, including the dataset
files and do-files, is available at
http://web.uni-bamberg.de/sowi/soziologie-i/eha/stata.
About the Authors
Hans-Peter Blossfeld is a professor of sociology and director of the
Institute for Family Research at Bamberg University. Former editor of
the European Sociological Review, he received his PhD in economics from
the University of Mannheim in 1984.
Katrin Golsch is an assistant professor of economics at the University
of Cologne. She received her PhD in sociology from Bielefeld University
in Germany in 2004 under the supervision of Hans-Peter Blossfeld. She
teaches a course on introduction to Stata.
Götz Rohwer is a professor of social research and statistics at the
Ruhr–University Bochum. He is the author of several books, including a
monograph on probability in the health sciences.
Table of contents
Preface
1 Introduction - 1.1 Causal Modeling and Observation Plans
1.1.1 Cross-Sectional Data
1.1.2 Panel Data
1.1.3 Event History Data
1.2 Event History Analysis and Causal Modeling
2 Event History Data Structures
- 2.1 Basic Terminology
2.2 Event History Data Organization
3 Nonparametric Descriptive Methods
- 3.1 Life Table Method
3.2 Product-Limit Estimation
3.3 Comparing Survivor Functions
4 Exponential Transition Rate Models
4.1 The Basic Exponential Model
4.1.1 Maximum Likelihood Estimation
4.1.2 Models without Covariates
4.1.3 Time-Constant Covariates
4.2 Models with Multiple Decisions
4.3 Models with Multiple Episodes
5 Piecewise Constant Exponential Models
- 5.1 The Basic Model
5.2 Models without Covariates
5.3 Models with Proportional Covariate Effects
5.4 Models with Period-Specific Effects 6 Exponential Models with Time-Dependent Covariates
- 6.1 Parallel and Interdependent Processes
6.2 Interdependent Processes: The System Approach
6.3 Interdependent Processes: The Causal Approach
6.4 Episode Splitting with Qualitative Covariates
6.5 Episode Splitting with Quantitative Covariates
6.6 Application Examples
7 Parametric Models of Time-Dependence - 7.1 Interpretation of Time-Dependence
7.2 Gompertz Models
7.3 Weibull Models
7.4 Log-Logistic Models
7.5 Log-Normal Models
8 Methods to Check Parametric Assumptions
- 8.1 Simple Graphical Methods
8.2 Pseudoresiduals
9 Semiparametric Transition Rate Models - 9.1 Partial Likelihood Estimation
9.2 Time-Dependent Covariates
9.3 The Proportionality Assumption
9.4 Baseline Results and Survivor Functions
9.5 Application Example
10 Problems of Model Specification
- 10.1 Unobserved Heterogeneity
10.2 Models with a Mixture Distribution
10.2.1 Models with a Gamma Mixture
10.2.2 Exponential Models with a Gamma Mixture
10.2.3 Weibull Models with a Gamma Mixture
10.3 Discussion
- References
Abouth the Authors
Index
© Copyright StataCorp LP 1996-2010.


|