|
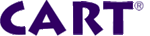
by 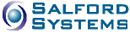
CART 6.0 ProEX Features
CART 6.0 ProEX, newly released for 2008, comes with
a huge list of new features that will help analysts work more rapidly
and guide their models to the best-performing trees. This is a dramatic
upgrade of our flagship product and is drawing rave reviews from our
customers. All of the new CART 6.0 ProEX features are explained in
detail in our feature matrix (PDF); some highlights are listed below:
Tree Controls
- Force splitters into nodes
- Confine select splitters to specific regions of a tree (Structured Tree™)
HotSpot Detector™
- Search data for ultra-high performance segments.
- HotspotDetector trees are specifically designed to
yield extraordinarily high-lift or high-risk nodes. The process focuses
on individual nodes and generally discards the remainder of the tree.
Train/Test Consistency Assessment
- Node-by-node summaries of agreement between train and test data on both class assignment and rank ordering of the nodes.
- Quickly identify ideally-performing robust trees.
Modeling Automation
- Automatically generate entire collections of trees exploring different control parameters.
- Nineteen automated batteries cover exploration of
multiple splitting rules, five alternative missing value handling
strategies, random selection of alternative predictor lists,
progressively smaller (or larger) training sample sizes, and much more.
Predictor Refinement
- Includes stepwise backwards predictor elimination
using any of three predictor ranking criteria (lowest variable
importance rank, lowest loss of area under the ROC curve, highest
variable importance rank).
Model Assessment via Monte Carlo Testing
- Measure possible overfitting with automated Monte Carlo randomization tests.
Constructed Features
- New tools for automatic construction of new features (as linear combinations of predictors).
- Identification of multiple lists of candidates allows
precise control over which predictors may be combined into a single new
feature.
Unsupervised Learning Mode
- Use Breiman's column scrambler to automatically
detect potential clusters with no need to scale data, address missing
values, or select variables for clustering.
© Copyright 2015 Salford-Systems Inc.


|
|